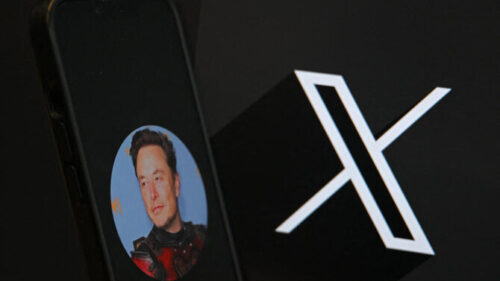
Elon Musk’s X fails bid to escape Australian fine
Elon Musk’s X fails bid to escape Australian fine Elon Musk’s X on Friday lost a legal bid to avoid a $417,000 fine levelled by
Statistics plays a crucial role in data science, providing the tools and techniques necessary to extract meaningful insights from data. In this tutorial, we will explore the fundamental concepts of statistics and how they are applied in data science.
Statistics is a branch of mathematics that deals with the collection, analysis, interpretation, presentation, and organization of data. It enables us to make informed decisions and predictions based on data.
In data science, statistics is used to:
R is a popular programming language for statistical analysis and data visualization. It provides a wide range of packages for statistical modeling and machine learning.
Regression analysis is a statistical method used to model the relationship between a dependent variable and one or more independent variables. It is commonly used for predicting outcomes and understanding the relationship between variables.
Bayesian statistics is a framework for incorporating prior knowledge or beliefs into statistical inference. It provides a way to update beliefs based on new evidence, making it particularly useful in situations with limited data.
Time series analysis is used to analyze time-stamped data to uncover patterns and trends over time. It is commonly used in forecasting and monitoring applications.
Statistics is a vast field with many advanced concepts that are essential for data scientists. By mastering these concepts and techniques, data scientists can gain deeper insights from data and build more accurate predictive models.
You must be logged in to post a comment.
WELCOME TO SOFTDOZE.COM
Softdoze.com is a technology-focused website offering a wide range of content on software solutions, tech tutorials, and digital tools. It provides practical guides, reviews, and insights to help users optimize their use of software, improve productivity, and stay updated on the latest technological trends. The platform caters to both beginners and advanced users, delivering useful information across various tech domains.
Elon Musk’s X fails bid to escape Australian fine Elon Musk’s X on Friday lost a legal bid to avoid a $417,000 fine levelled by
Facebook, one of the original social media networks Facebook, one of the original social media networks, has become known as the platform of parents and
Bangabandhu Sheikh Mujibur Rahman Bangabandhu Sheikh Mujibur Rahman Born: March 17, 1920, Tungipara, Gopalganj, British India (now Bangladesh) Died: August 15, 1975, Dhaka, Bangladesh Role:
হারানো-নষ্ট হয়ে যাওয়া ড্রাইভিং লাইসেন্স উত্তোলন হারানো ড্রাইভিং লাইসেন্স উত্তোলন করার উপায়। আমাদের মাঝে অনেকেই আছেন যারা ড্রাইভিং লাইসেন্স হারিয়ে ফেলছেন অথবা নষ্ট করে ফেলছেন।
Submit a Comment